Model Compression Overview and Resources
此NOTE主要记录一些关于model compression 方面的overview和一些不错的入门资源、survey and papers.
- Model pruning(模型剪枝): removes less important parameters
- Weight Quantization: uses fewer bits to represent the parameters
- Parameter sharing
- Knowledge distillation(知识蒸馏): trains a smaller student model that learns from intermediate outputs from the original model.
- Module replacing / Dynamic Computation: Can network adjust the computation power it need?
Videos
- 知识蒸馏简述–起源、改进与研究现状【截至2020年3月22日】
- 模型压缩Network Compression方法补充 NOTE: 1的延续
- 网络压缩和知识蒸馏-李宏毅
- 【深度学习的模型压缩与加速】台湾交通大学 張添烜教授
Slides
Overview: https://slides.com/arvinliu/model-compression
Deep Mutual Learning https://slides.com/arvinliu/kd_mutual
Blogs
- 深度学习模型压缩与加速综述 LINK
- 知识蒸馏是什么?一份入门随笔 LINK
- 知识蒸馏(Knowledge Distillation)简述(一)LINK
- Mutual Mean-Teaching:为无监督学习提供更鲁棒的伪标签 LINK
Papers and Surveys
Papers:
- Knowledge Distillation 2015 LINK 知识蒸馏开山之作
- The Lottery Ticket Hypothesis: Finding Sparse, Trainable Neural Networks 2019 LINK
- Rethinking the Value of Network Pruning 2019 LINK
- BinaryConnect: Training Deep Neural Networks with binary weights during propagations 2015 LINK
- Binarized Neural Networks: Training Deep Neural Networks with Weights and Activations Constrained to +1 or -1 2016 LINK
- XNOR-NET 2016 LINK
- MobileNets 2017 LINK
- SqueezeNet 2016 LINK
- Multi-Scale Dense Networks for Resource Efficient Image Classification 2018 LINK
- Label Refinery: Improving ImageNet Classification through Label Progression 2018 LINK
- Deep Mutual Learning 2018 LINK
- Born Again Neural Networks 2018 LINK
- Improved Knowledge Distillation via Teacher Assistant 2019 LINK
- FITNETS: HINTS FOR THIN DEEP NETS 2015 LINK
- Relational Knowledge Distillation 2019 LINK
- Similarity-Preserving Knowledge Distillation 2019 LINK
- Pruning Filters for Efficient ConvNets 2017 LINK
- Learning Efficient Convolutional Networks Through Network Slimming 2017 LINK
- Filter Pruning via Geometric Median for Deep Convolutional Neural Networks Acceleration 2019 LINK
- Network Trimming: A Data-Driven Neuron Pruning Approach towards Efficient Deep Architectures 2016 LINK
- Deconstructing Lottery Tickets: Zeros, Signs, and the Supermask 2019 LINK
Surveys:
Overview
model compression最主要包含以下四类大方法,每一类并不是独立的,是可以交替和交叉使用的。
如下图所示:
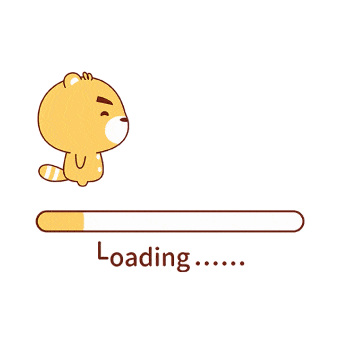
Network Pruning
主要思想为:将Network不重要的weight或neuron删除后,再重新训练一次。
General Reason:尤其在深度学习领域,网路很深,模型参数极多,存在很多冗杂的参数。
应用:所有有神经网络的地方基本都可以使用。
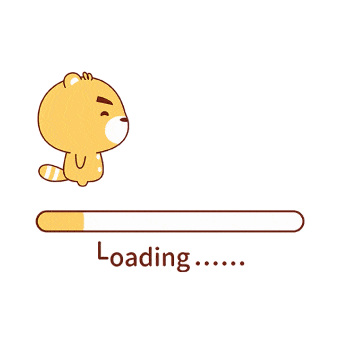
其整体流程大概可以如上图所示。
NOTE:这里如何计算weight或neuron的重要程度有各种不同的方法,e.g. L2, L1 Norm,the number of times it wasn’t zero on a given data set ……
可以看到,这是一个iteration的过程。
Why Pruning
为什么要做network Pruning? 而不是直接在一个小的model上学习?
经验上来讲:It is widely known that smaller network is more difficult to learn successfully. 即:大模型的训练往往比小模型更加简单,即更容易跳过一些local minimum。
当然也有一些关于这方面的理论,如:
- Lottery Ticket Hypothesis LINK
- Larger network is easier to optimize? [LINK](https://www.youtube.com/watch?v=_VuWvQU
MQVk) - Rethinking the Value of Network Pruning LINK NOTE: Lottery Ticket Hypothesis的反例
基本上来讲network Pruning可以分为:
对于weight 做pruning
这种方法的最大问题是导致不方便implement!!!因为现在GPU加速都是使用矩阵运算,这样容易使网络结构变的不规则。导致无法Speed Up甚至会出现pruning后速度反而下降的情况。
在Practice中这类weight pruning常常就简单的将要pruning的weight设置为0,这样显然并没有办法对于模型的体积进行压缩。
对于Neuron 做pruning
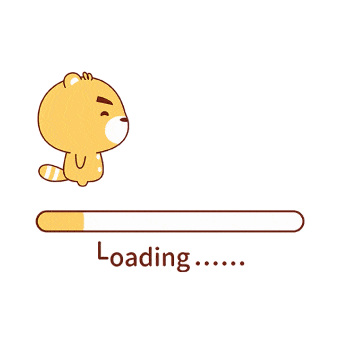
可以看到,在pruning后,整个网络仍然是regular的,可以继续使用GPU进行加速。实践中比较常用。
What is important? How to evaluate importance?
如何来衡量一个weight or neuron的importance
- evaluate by weight(看大小)
- evaluate by activation
- evaluate by gradient
After that?
- Sort by importance and prune by rank
- prune by handcrafted threshold
- prune by generated threshold
**Threshold or Rank? **
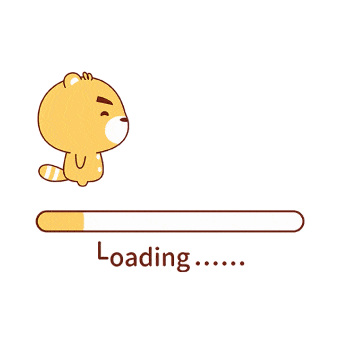
Evaluate Importance
这部分主要关注evaluate weight.
sum of L1 norm(也可以是其他范数)
这种直接使用norm的方法一般如下(对于CNN而言):
将卷积的filter排列为矩阵,每个filter的channel拼成一行,之后对每一行算norm,根据此norm来选择去掉哪些filter.
理想的norm分布应该如下图所示,即有:
- norm非常接近0的部分
- 整体是一个比价均匀,而且有较大方差的分布
我们就想要prune掉norm接近0的部分。
而真实的分布往往并不尽如人意。
- 方差很小,此时很难选取一个合适的threshold。
- 没有接近0的部分,不接近0,很难从norm的角度说明一个filter他trivial。
FPGM(Filiter Pruning via Gemetirc Media 2019): 大的norm一定important?小的norm的一定trivial?用Gemetirc Media来解决这个问题。(解决hazard of pruning by norm)如下图所示。
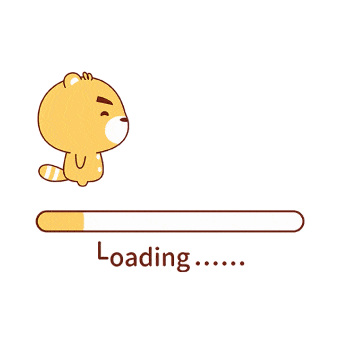
Evaluate By BN(Batch Norm) Network Slimming
- 根据BN的γ来判断是否pruning
- 而往往γ的分布并不好,我们需要做一些penalty。让这个分布更容易被筛选。
Eval by 0s after ReLU - APoZ(Average Percentage of Zeros)
Some theory about Network Pruning
Lottery Ticket Hypothesis
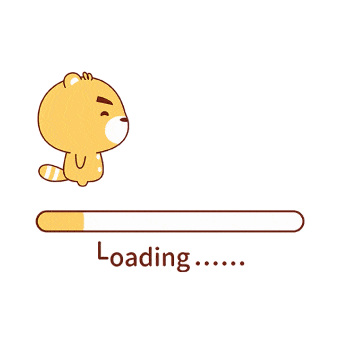
Rethinking the Value of Network Pruning
Rethinking VS Lottery Ticket
Rethinking: 一种neuron pruning or structural pruning
Lottery Ticket: 一种 weight pruning,且要求learning rate要小。
Knowledge Distillation
主要思想为:利用一个已经训练好的大model做teacher,来训练小model(student).
最核心的思路为下图所示:
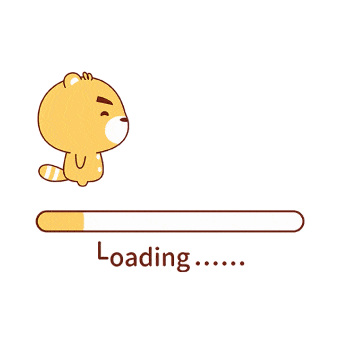
对于Knowledge Distillation(下文简称为KD)的分类,基本上可以按照Distill What来分类。
- Logits 即:网络的输出值,一个label的概率分布
- 直接一对一匹配logits
- 以batch为学习单位来学习其中的logits distillation
- ……
- Feature 即:网络每层中的中间值
- 直接一对一匹配每层的中间值
- 学习teacher网络中feature是如何转换的
The Power of Soften Label
对于分类任务,我们模型的输出并不是想真实的label中一样,是一个one-hot encoding,而是一组在许多label上都用几率的一组概率分布。可以直观的看到,这个模型的输出,相比于真实的label包含了更多的信息,甚至包含了类别间的relationship. 现在有一类研究方向就是在训练时不适用这种one-hot encoding,而是研究如何产生包含更多信息的Soften Label。
例如这篇Label Refinery: Improving ImageNet Classification through Label Progression LINK 之后写这篇文章的总结和介绍。
Logits Distillation
本质就是:通过soft target让小model可以学到class之间的关系。
一些比较有趣的Work:
- Deep Mutual Learning
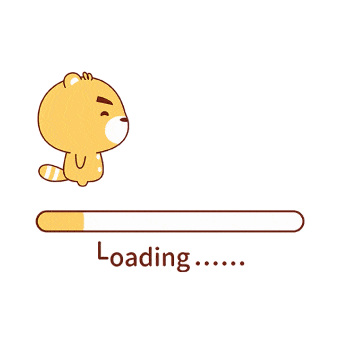
- Born Again Neural Networks
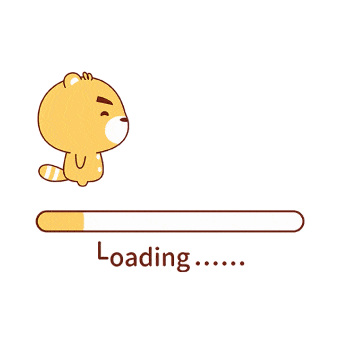
显然,这其中存在着一些问题,可能因为teacher模型的模型能力过强,而小模型的能力不足,导致无法很好地直接学习。
其中的一种很有趣的解决方法就是,向我们上课一样,引入TA。TA模型的能力介于teacher和student之间。这样可以避免缩小模型间的差距。下图即为:Improved Knowledge Distillation via Teacher Assistant 2020这篇paper的想法。
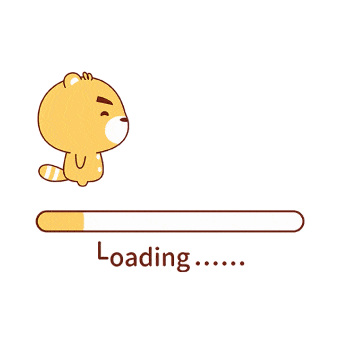
Feature Distillation
不再是直接根据logits来学习,而是学习网络中的中间features。
其代表方法有:
- FitNet: 先让学生学习如何产生teacher的中间feature,以后再使用标准的KD。NOTE:框架越相似,效果越好。
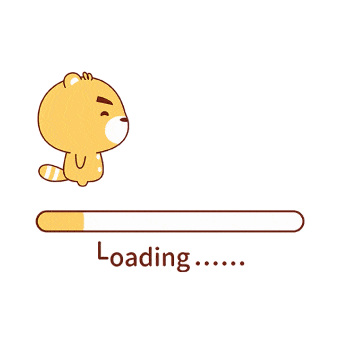
该方法存在两大问题:
- model capacity is different. 显然如果大模型很复杂,可能小模型的中间部分无法学习到大模型的复杂映射。
- redundance in teacher feature. 这个是很直观的,对于复杂的模型,这个feature中并不是所有的部分都是起作用的,这些对于小模型来讲是一个学习的负担。
解决上述问题的方法可以是对于大模型的每一个feature map做一个知识蒸馏,目的就是在压缩feature的同时也降低了redundance.
另外一种的解决方法就是使用Attention,告诉student model中的feature map(主要指CNN)中那些part是最重要的。
下图是一种简单计算attention的方法,就是将filter对应产生的out dim做一个压缩。
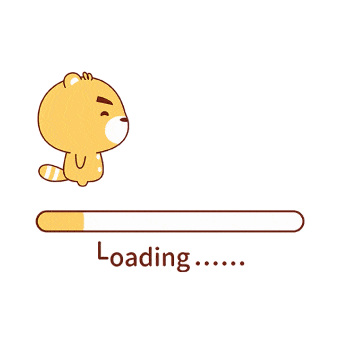
Relational Distillation: Learn from Batch
前面的不论是logit KD还是feature KD,都是对于每一个sample来学习的(即:individual KD),这类Relational Distillation关注的则通过一个batch来distillation batch之间sample的关系。
下图是这individual KD与Relational KD的paradigm的对比。
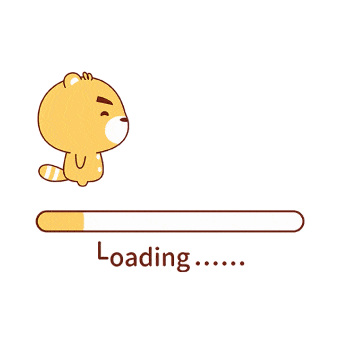
而衡量sample之间的relation 可以用以下的两种描述角度:
- Distance-wise KD: 使用L2 distance来描述。
- Angle-wise KD: 使用cosin similarity来描述。
该方法是使用logits来做KD的,自然也可以使用features来做KD。这就有了:Similarity-Preserving Knowledge Distillation这篇文章。
Parameter Quantization
将原本神经网络中数据的存储单位float32/64压缩为更小的单位,例如8位。
应用:对于所欲已经train好的模型使用,或者边train边让模型去做quantize。
基本来讲大致分为以下方法:
- Using less bits to represent a value
- Weight clustering
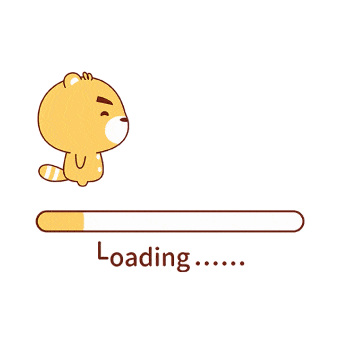
- Represent frequent clusters by less bits, represent rare clusters by more bits. e.g. Huffman encoding
其中一类是使用Binary Weight。(从某种角度上,也是一种正则化的方法)
Architecture Design
方法:利用更少的参数来实现原本某些layer效果。
例如对于全连接层:
我们可以将原本的 N到M维的映射 变为 N->K->M的映射。从矩阵乘法的角度来看,这可以看作一种Low rank approximate. 这种方法也可以极大的减少全连接层的参数数量。
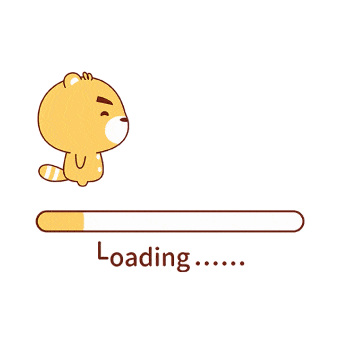
对于卷积层:可以使用Depthwise Separable Convolution(这也是大名鼎鼎的mobile net使用的方法)
即将原本一步的卷积运算变为两个卷积运算,如下图所示。
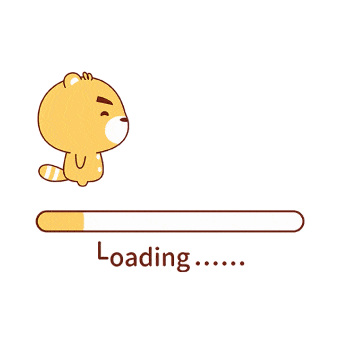
其他的一些经典的这类design有:
- MobileNet
- SqueezeNet
- Xception
- ShuffleNet
Dynamic Computation
核心思想:Can network adjust the computation power it need?
即:若此时计算资源充分,使用大模型;若不足,使用较小的模型。
可能的解决方法:
Train multiple classifiers
Classifiers at the intermedia layer 例如 Multi-Scale Dense Networks LINK